Empowering healthcare with AI: LungDiag NLP system transforms respiratory disease diagnosis
In a recent study posted to the Preprints with The Lancet* SSRN preprint server, researchers in Guangzhou, China, developed a natural language processing (NLP) or artificial intelligence (AI) -based diagnostic system, LungDiag, to diagnose respiratory diseases using electronic health records (EHRs) from multiple hospitals in China.
Additionally, they compared LungDiag's performance externally with physicians and ChatGPT 4.0.
Study: LungDiag: Empowering Artificial Intelligence for Respiratory Disease Diagnosis Through Electronic Health Records. Image Credit: SomYuZu/Shutterstock.com
*Important notice: SSRN publishes preliminary scientific reports that are not peer-reviewed and, therefore, should not be regarded as conclusive, guide clinical practice/health-related behavior, or treated as established information.
Background
The burden of respiratory diseases is increasing globally. Many of these diseases share symptoms, thus, are difficult to diagnose, which, in turn, hampers the initiation of timely treatment. As a result, patient outcomes are poor, and healthcare cost rises.
Thus, the Forum of International Respiratory Societies (FIRS) has made disease prevention and early diagnosis of respiratory diseases a research priority.
Due to diverse data and intricate structures, EHRs are highly unstructured. Its structured part, though relatively small, comprises patient diagnoses, prescriptions, and laboratory test results, whereas clinical notes constitute its unstructured part.
Globally, EHRs have been adopted as 'big data' sources of healthcare data. Thus, if an NLP algorithm could help extract structured clinical phenotype from EHR data, it could improve respiratory disease diagnosis.
An intelligent diagnostic system utilizing EHRs is an urgent, unmet need for diagnosing specific respiratory diseases early.
About the study
In the present retrospective study, researchers gathered EHRs of inpatients with respiratory disease(s) from the First Affiliated Hospital of Guangzhou Medical University for training LungDiag and its internal testing.
They collected these EHRs between November 1, 2012, and October 30, 2019, and EHRs of in-hospital patients from three other hospitals in China for external testing of LungDiag.
LungDiag performed two main functions: first, it used NLP to identify distinct clinical phenotypes from EHRs; second, it employed machine learning to classify respiratory diseases based on known fine-grained clinical attributes.
The NLP algorithm used in this study required manual annotation and used deep learning techniques for standardization of clinical features\phenotype in EHRs. Likewise, the team used a Bi-LSTM-CRF model with a "BIO" tagging schema to label sequences of six phenotypic entities, viz. disease symptoms, names, related quantitative\qualitative test results, imaging results, medications used, and surgeries done.
Two human clinicians extracted phenotypic features from medical textbooks and clinical guidelines to further refine those extracted by the study model. Finally, the team used Unified Medical Language System or UMLS to extract 442 clinical features associated with respiratory disease diagnosis and standardize them into 252 clinical features.
Results
The training dataset comprised 31,267 EHRs of 21,490 male and 9,777 female patients with a median age of 64. Per EHR data, ten types of respiratory diseases constituted 80.7% of the diseases in the EHRs, and chronic obstructive pulmonary disease, or COPD, was the most prevalent. The external testing dataset comprised 1,142 additional EHRs.
The LungDiag AI-based system recognized entities within EHRs and extracted and interpreted major clinical phenotypes with precision and recall, thereby facilitating disease classification. Its performance highlighted how automated systems could navigate the complexity of EHRs seamlessly while streamlining the identification of clinical phenotypes, which is a tedious task for healthcare personnel.
The LungDiag also outperformed physicians achieving F1 scores for top one and top three diagnoses at 0.745 and 0.927, respectively, and ChatGPT 4.0. ChatGPT achieved average F1 scores similar to human physicians but not LungDiag. However, clinical notes processing and securing a patient's data privacy using LungDiag remain challenging.
The ablation experiment results confirmed that fine-grained phenotypic features exhibited superior diagnostic performance and enabled AI to learn more features. Relative to coarse-grained phenotypic features, fine-grained features had higher average precision, recall, and F1 scores.
Accordingly, these scores increased by 2%, 4%, and 3.3% and 2.3%, 4%, and 3.4% for top one and top three diagnoses, respectively.
Conclusions
The system used in this study holds significant potential to aid the diagnosis of respiratory diseases. It could also help medical professionals manage voluminous inpatient records and offer clinical advice despite diagnostic uncertainties.
Performance-wise, LungDiag standardized all discharge diagnoses into ten types of respiratory diseases and attained an average precision, recall, and F1 score of 0.883, 0.819, and 0.899, respectively, in recognizing all six phenotypic entities.
It also demonstrated high accuracy in respiratory disease classification, with a median precision of 0.763, recall of 0.677, and F1 score of 0.711 for the top one and 0.965, 0.897, and 0.927 for the top three diagnoses.
Overall, LungDiag exhibited superior performance and exceptional accuracy and recall.
*Important notice: SSRN publishes preliminary scientific reports that are not peer-reviewed and, therefore, should not be regarded as conclusive, guide clinical practice/health-related behavior, or treated as established information.
- Preliminary scientific report.
Liang, H. et al. (2023) "LungDiag: Empowering Artificial Intelligence for Respiratory Disease Diagnosis Through Electronic Health Records". SSRN. doi: 10.2139/ssrn.4502081. https://papers.ssrn.com/sol3/papers.cfm?abstract_id=4502081
Posted in: Device / Technology News | Medical Science News | Medical Research News | Medical Condition News | Disease/Infection News | Healthcare News
Tags: Artificial Intelligence, Chronic, Chronic Obstructive Pulmonary Disease, Deep Learning, Diagnostic, Healthcare, Hospital, Imaging, Laboratory, Language, Machine Learning, Phenotype, Research, Respiratory, Respiratory Disease
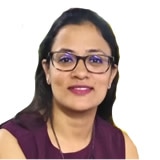
Written by
Neha Mathur
Neha is a digital marketing professional based in Gurugram, India. She has a Master’s degree from the University of Rajasthan with a specialization in Biotechnology in 2008. She has experience in pre-clinical research as part of her research project in The Department of Toxicology at the prestigious Central Drug Research Institute (CDRI), Lucknow, India. She also holds a certification in C++ programming.