Machine learning-based preeclampsia risk prediction
In a recent study posted to the medRxiv* preprint server, researchers developed a machine-learning-based model using the Medical Record Longitudinal Information AI System (MERLIN) platform for longitudinal preeclampsia risk estimation.
Study: An Interpretable Longitudinal Preeclampsia Risk Prediction Using Machine Learning. Image Credit: Yuriy K/Shutterstock.com
*Important notice: medRxiv publishes preliminary scientific reports that are not peer-reviewed and, therefore, should not be regarded as conclusive, guide clinical practice/health-related behavior, or treated as established information.
Background
Preeclampsia is a specific condition that causes hypertension and proteinuria after 20.0 gestational weeks, complicating pregnancies and contributing to maternal fatalities.
Proteinuria, increased liver enzymes, lung edema, convulsions, and death can all occur due to increasing end-organ damage.
Preeclampsia is the major cause of iatrogenic premature deliveries with accompanying infant morbidity and death since there is no definite cure.
Despite substantial clinical research, existing prediction methods fail to detect preeclampsia-risk individuals. Furthermore, nothing is known about the risk trajectory or the pace of change in risk during pregnancy.
About the study
In the present study, researchers devised and validated the MERLIN machine learning tool to estimate preeclampsia risk throughout pregnancy.
The study included a large group of women who gave birth at two hospitals for tertiary care and six centers for community care in New England between February 2015 and June 2023.
Sociodemographics, family history, clinical diagnoses, vital signs, and laboratory reports were analyzed. The team included only deliveries with ≥1.0 visits with recorded data before 14.0 weeks of pregnancy. Eight datasets were developed at weeks 14.0, 20.0, 24.0, 28.0, 32.0, 36.0, and 39.0 of gestation and hospitalization for childbirth.
The team developed a combination of blood pressure measurements, ICD codes, and laboratory results to determine the preeclampsia phenotype in the datasets. Linear regression, xgboost random forest classifiers, deep neural networks (DNN), and elastic net models were used to develop the tool and evaluate its performance.
The area under the curve (AUC) metric was used to estimate the predictive power of the tool. The model was validated using the electronic health records of 400.0 preeclampsia patients, reviewed by two clinical experts.
The training and testing datasets comprised 80% and 20% of cases, respectively. The team performed a 5.0-fold cross-validation over the training set to ascertain hyperparameters, following which the most appropriate combination was used to determine the testing metrics.
Shapley values were used to explain model outputs. In addition, databases such as the Web of Science, MEDLINE, and PubMed were searched from the study’s inception through 1 May 2023.
Results
Among 120,757 individuals, the preeclampsia incidence was 5.7% (6,920 individuals). The AUC values for the model ranged between 0.7 and 0.9, which was validated externally. The associations between a few variables were non-linear and complex; additionally, the relative statistical significance of risk estimators varied during the pregnancy period.
In comparison to the standard for predicting preeclampsia risk in the first trimester, the machine learning-based tool detected 49% more patients with preeclampsia risk.
In addition, using the xgboost model predictions at 14.0 weeks on the testing dataset, 25% (n=5,624) individuals would be eligible for aspirin prophylaxis, whereas 15% (n=3,295) would be eligible using the American College of Obstetricians and Gynecologists (ACOG) criteria.
Using the novel model, an additional 2,329 individuals would have been eligible. If aspirin prophylaxis could prevent 62.0% of preeclampsia among high-risk individuals, an additional 28 cases (a total of 66 cases) of early-onset preeclampsia among every 10,000 pregnant women would have the potential to be prevented with updated risk prediction.
Testing different predictive model types involving deep and machine learning showed high estimation power.
Compared to normotensive individuals, those with a preeclampsia diagnosis included a significantly higher percentage of Blacks (17.0% versus 9.0%) and Hispanics (19.0% versus 15.0%), respectively.
Additionally, preeclampsia patients had an increased likelihood of familial hypertension, higher maximal diastolic and systolic blood pressures, and higher weight gain during pregnancy than non-preeclampsia individuals.
At 14.0 weeks, the most estimative features were long inter-pregnancy intervals and chronic hypertension. With increased gestational age, diastolic and systolic blood pressure, laboratory reports, and vital signs were major contributors.
Further, proteinuria was unlikely to be related to preeclampsia diagnosis if the maximal systolic blood pressure during pregnancy was below 140.0 mm of Hg; however, above the threshold, proteinuria became highly predictive.
Individuals under 20 and above 35 years were at increased risk of preeclampsia. A higher erythrocyte count in the second trimester was related to higher preeclampsia risks.
In total, 13 studies were identified that incorporated machine learning to predict preeclampsia risk using clinical parameters, among which six included biological markers such as the uterine artery pulsatility index, serological placental growth factor (PIGF), and pregnancy-related serum protein A; two studies included diverse groups of >100,000 individuals; and two relevant studies conducted longitudinal estimations using electronic health records.
However, most studies had limited depth, raised data leakage concerns, were overfitted, and lacked generalizability.
Based on the study findings, the risk prediction model for preeclampsia can aid in the early identification of high-risk individuals, enabling longitudinal risk assessments during pregnancy. Accurate risk prediction can benefit clinical treatment, aspirin prophylaxis, surveillance, and care escalation. Artificial intelligence can enhance perinatal care and reduce iatrogenic preterm births.
*Important notice: medRxiv publishes preliminary scientific reports that are not peer-reviewed and, therefore, should not be regarded as conclusive, guide clinical practice/health-related behavior, or treated as established information.
- Preliminary scientific report.
Eberhard, B. et al. (2023) "An Interpretable Longitudinal Preeclampsia Risk Prediction Using Machine Learning". medRxiv. doi: 10.1101/2023.08.16.23293946. https://www.medrxiv.org/content/10.1101/2023.08.16.23293946v1
Posted in: Device / Technology News | Medical Science News | Medical Research News | Medical Condition News | Healthcare News
Tags: Artificial Intelligence, Aspirin, Blood, Blood Pressure, Childbirth, Chronic, Edema, Growth Factor, Laboratory, Liver, Machine Learning, Phenotype, Pregnancy, Prophylaxis, Protein, Proteinuria, Research
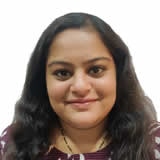
Written by
Pooja Toshniwal Paharia
Dr. based clinical-radiological diagnosis and management of oral lesions and conditions and associated maxillofacial disorders.