Researchers from HSE University and the Moscow State University of Medicine and Dentistry have developed a machine learning model that can predict the word about to be uttered by a subject based on their neural activity recorded with a small set of minimally invasive electrodes. The paper 'Speech decoding from a small set of spatially segregated minimally invasive intracranial EEG electrodes with a compact and interpretable neural network' has been published in the Journal of Neural Engineering . The research was financed by a grant from the Russian Government as part of the 'Science and Universities' National Project.
Millions of people worldwide are affected by speech disorders limiting their ability to communicate. Causes of speech loss can vary and include stroke and certain congenital conditions.
Technology is available today to restore such patients' communication function, including 'silent speech' interfaces which recognize speech by tracking the movement of articulatory muscles as the person mouths words without making a sound. However, such devices help some patients but not others, such as people with facial muscle paralysis.
Speech neuroprostheses-;brain-computer interfaces capable of decoding speech based on brain activity-;can provide an accessible and reliable solution for restoring communication to such patients.
Unlike personal computers, devices with a brain-computer interface (BCI) are controlled directly by the brain without the need for a keyboard or a microphone.
A major barrier to wider use of BCIs in speech prosthetics is that this technology requires highly invasive surgery to implant electrodes in the brain tissue.
The most accurate speech recognition is achieved by neuroprostheses with electrodes covering a large area of the cortical surface. However, these solutions for reading brain activity are not intended for long-term use and present significant risks to the patients.
Researchers of the HSE Centre for Bioelectric Interfaces and the Moscow State University of Medicine and Dentistry have studied the possibility of creating a functioning neuroprosthesis capable of decoding speech with acceptable accuracy by reading brain activity from a small set of electrodes implanted in a limited cortical area. The authors suggest that in the future, this minimally invasive procedure could even be performed under local anaesthesia. In the present study, the researchers collected data from two patients with epilepsy who had already been implanted with intracranial electrodes for the purpose of presurgical mapping to localise seizure onset zones.
The first patient was implanted bilaterally with a total of five sEEG shafts with six contacts in each, and the second patient was implanted with nine electrocorticographic (ECoG) strips with eight contacts in each. Unlike ECoG, electrodes for sEEG can be implanted without a full craniotomy via a drill hole in the skull. In this study, only the six contacts of a single sEEG shaft in one patient and the eight contacts of one ECoG strip in the other were used to decode neural activity.
The subjects were asked to read aloud six sentences, each presented 30 to 60 times in a randomised order. The sentences varied in structure, and the majority of words within a single sentence started with the same letter. The sentences contained a total of 26 different words. As the subjects were reading, the electrodes registered their brain activity.
Neuroscience eBook
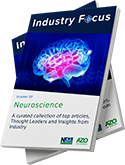
This data was then aligned with the audio signals to form 27 classes, including 26 words and one silence class. The resulting training dataset (containing signals recorded in the first 40 minutes of the experiment) was fed into a machine learning model with a neural network-based architecture. The learning task for the neural network was to predict the next uttered word (class) based on the neural activity data preceding its utterance.
In designing the neural network's architecture, the researchers wanted to make it simple, compact, and easily interpretable. They came up with a two-stage architecture that first extracted internal speech representations from the recorded brain activity data, producing log-mel spectral coefficients, and then predicted a specific class, ie a word or silence.
Thus trained, the neural network achieved 55% accuracy using only six channels of data recorded by a single sEEG electrode in the first patient and 70% accuracy using only eight channels of data recorded by a single ECoG strip in the second patient. Such accuracy is comparable to that demonstrated in other studies using devices that required electrodes to be implanted over the entire cortical surface.
The resulting interpretable model makes it possible to explain in neurophysiological terms which neural information contributes most to predicting a word about to be uttered. The researchers examined signals coming from different neuronal populations to determine which of them were pivotal for the downstream task. Their findings were consistent with the speech mapping results, suggesting that the model uses neural signals which are pivotal and can therefore be used to decode imaginary speech.
Another advantage of this solution is that it does not require manual feature engineering. The model has learned to extract speech representations directly from the brain activity data. The interpretability of results also indicates that the network decodes signals from the brain rather than from any concomitant activity, such as electrical signals from the articulatory muscles or arising due to a microphone effect.
The researchers emphasize that the prediction was always based on the neural activity data preceding the utterance. This, they argue, makes sure that the decision rule did not use the auditory cortex's response to speech already uttered.
The use of such interfaces involves minimal risks for the patient. If everything works out, it could be possible to decode imaginary speech from neural activity recorded by a small number of minimally invasive electrodes implanted in an outpatient setting with local anesthesia."
Alexey Ossadtchi, leading author of the study, director of the Centre for Bioelectric Interfaces of the HSE Institute for Cognitive Neuroscience
National Research University Higher School of Economics
Petrosyan, A., et al. (2022) Speech decoding from a small set of spatially segregated minimally invasive intracranial EEG electrodes with a compact and interpretable neural network. Journal of Neural Engineering. doi.org/10.1088/1741-2552/aca1e1.
Posted in: Device / Technology News | Medical Science News
Tags: Auditory Cortex, Brain, Cortex, Dentistry, Electrode, Epilepsy, Machine Learning, Medicine, Muscle, Neuroscience, Paralysis, Research, Seizure, Speech, Stroke, Surgery, Technology
Source: Read Full Article