In a recent study posted to the medRxiv* preprint server, an interdisciplinary team of researchers from the United States (US) assessed predictions of the laboratory-confirmed coronavirus disease 2019 (COVID-19) incident rates (IRs) through severe acute respiratory syndrome coronavirus 2 (SARS-CoV-2) ribonucleic acid (RNA) concentrations in the wastewater solids using robust distributed lag models (DLMs).

The team analyzed the effect of sampling frequency on the prediction error of IRs by using reduced sampling across different sewer sheds of varying sizes and features.
Background
During the COVID-19 pandemic, researchers across the globe have used wastewater-based epidemiology and monitored SARS-CoV-2 RNA concentrations in wastewater to predict COVID-19 incident rates in the community.
The DLM uses the effect of an explanatory variable occurring over time rather than once on the dependent variable. DLM has been applied in previous studies of COVID-19 wastewater surveillance but has not been used to identify optimum sampling frequencies nor the effect of emerging SARS-CoV-2 variants on the association between COVID-19 cases and SARS-CoV-2 RNA concentrations in wastewater.
The present study was conducted to estimate whether surveillance of wastewater could aid in the prediction of the real-time COVID-19 incidence rates using a regression model scaled to considerable and cost-effective sampling frequencies.
Study design
The study analyzed four publicly owned treatment works (POTWs) in California. Two of these POTWs served Santa Clara County (SJ and PA) population, and the remaining each POTWs served as a part of Sacramento County (Sac), SAN Mateo County (PA), and Yolo County (Dav). Approximately 50ml of wastewater solid samples were collected daily from each POTW between November 2020 and September 2021.
They used a high-throughput procedure consisting of automatic equipment and a liquid handling robot to measure the concentration of SARS-CoV-2 nucleocapsid (N) gene and pepper mild mottle virus (PMMoV) RNA with a bovine coronavirus (BCoV) as an external control.
The team down-sampled the datasets collected daily for N/PMMoV at each POTW to four lower sampling frequencies – once every two days, three days, four days, and weekly for model fitting. COVID-19 incident cases from each sewer shed were collected from georeferenced home addresses and delineated using geographic information system (GIS) shapefiles specific to the POTWs. COVID-19 incident rates were used as a dependent variable and calculated by the population estimated in each sewer shed.
Different regression models were fitted in the datasets sampled daily for each POTW like linear model (Eq1), DLM (Eq 2), and Eq 2 variations with percentage Delta as an explanatory variable. For reduced sampling frequencies, the team fitted both linear and DLM models for each POTW, resulting in a DLM with two predictors that fit each sampling frequency.
The researchers predicted the model performance by calculating the root mean square error (RMSE) for the in-sample (11 May 2020 to 19 July 2021) and out-of-sample period (20/07/2021 to 15/9/2021) and reported as IR (cases/100000).
Findings
In the four POTWs studied, the SARS-CoV-2 RNA N gene and PMMoV range were non-detected at 3.71×106 cp/g and 7.12×107 to 3.74×1010 cp/g. The maximum and minimum concentrations of N/PMMoV and SARS-CoV-2 RNA N gene were observed before the Delta surge.
The researchers selected the most effective model out of the candidate models using the data of N/PMMoV during the in-sample period for Eqs 1-2 and the whole sample period for Eqs 3-4. Based on the Bayesian Information Criterion (BIC), model fit in SJ Eq2 with U=3 was selected over the linear model. Notably, across all POTWs, the coefficient estimates of explanatory variables were significantly different from 0 and positive, reflecting that a higher log10 N/PMMoV was related to an increased log10 IR.
Linear and DLMs models were fitted using data of reduced sampling frequency of wastewater across the four POTWs. DLMs were preferred over the linear model for each sampling frequency based on increased in-sample R2 values. Between the DLMs and linear models, the BIC difference was <1.0 showing no preference. Except for the Sac weekly model, coefficient estimates for the regressors were positive and differed significantly from 0.
The team observed the highest increase in immediate impact effect only in SJ (t=0) for DLM fits across reduced sampling frequencies compared to the Dav, PA, and Sac. Linear regression coefficients across four POTWs, for N/PMMoV daily sampling ranged between 0.51 to 0.84. For daily sampling DLMs, coefficient estimates were identical to standard errors, which reduced sampling frequency.
The researchers noted a good model performance for out-of-sample. For SJ and PA, the RMSE for out-of-sample was reduced compared to in-sample, while for DA there was an increase of 3 cases/100000. Also, out-of-sample Sac RMSE was higher by 10 cases/100,000 than the in-sample RMSE.
For the model fit using the wastewater data at reduced sampling frequency across POTWs, the median RMSEs for out-of-sample were majorly 3 cases/100,000 higher compared to the in-sample median RMSEs, except for Sac with the highest difference of approximately 7 cases/100,000.
Across POTWs, a surge was observed in DLMs captured in in-sample and out-of-sample as predicted by IR traces. When reduced sampling frequency was used to predict IR, there was a major variation in prediction depending on the specific sample days.
Notably, across all POTWS, the RMSE of the model based on daily sampling was less than the RMSEs of the reduced frequency model in both in-sample and out-of-sample. The researchers observed that during the in-sample period, when daily sampling was decreased to weekly sampling, the maximum RMSE was increased by 20 cases/100,000 for SJ followed by 16, 8, and 4 cases/100,000 for Dav, Sac, and PA, respectively. While sampling once every two, three, and four days, the highest increase in RMSE from daily sampling was approximately 7 cases/100,000.
Conclusion
The findings of the study revealed that DLM had strong predictive power to estimate COVID-19 incident rates in California through wastewater surveillance of SARS-CoV-2 N genes. The DLMs monitored the surge and fall of COVID-19 cases during the Delta variant and other emerging variants using a model fit.
The study illustrated that on reduction of sampling frequency to once every four days and weekly, real-time prediction of COVID-19 IRs was feasible with fewer errors even with circulating SARS-CoV-2 variants.
*Important notice
medRxiv publishes preliminary scientific reports that are not peer-reviewed and, therefore, should not be regarded as conclusive, guide clinical practice/health-related behavior, or treated as established information.
-
Schoen, M. et al. (2022) "SARS-CoV-2 RNA wastewater settled solids surveillance frequency and impact on predicted COVID-19 incidence using a distributed lag model". medRxiv. doi: 10.1101/2022.02.21.22270864. https://www.medrxiv.org/content/10.1101/2022.02.21.22270864v1
Posted in: Medical Science News | Medical Research News | Disease/Infection News
Tags: Coronavirus, Coronavirus Disease COVID-19, covid-19, Epidemiology, Frequency, Gene, Genes, Laboratory, Liquid Handling, Pandemic, Respiratory, Ribonucleic Acid, RNA, SARS, SARS-CoV-2, Severe Acute Respiratory, Severe Acute Respiratory Syndrome, Syndrome, Virus
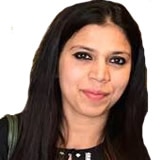
Written by
Sangeeta Paul
Sangeeta Paul is a researcher and medical writer based in Gurugram, India. Her academic background is in Pharmacy; she has a Bachelor’s in Pharmacy, a Master’s in Pharmacy (Pharmacology), and Ph.D. in Pharmacology from Banasthali Vidyapith, Rajasthan, India. She also holds a post-graduate diploma in Drug regulatory affairs from Jamia Hamdard, New Delhi, and a post-graduate diploma in Intellectual Property Rights, IGNOU, India.
Source: Read Full Article